Summary: Expanding on our earlier analysis, we explored how a government agency could use a large language model (LLM) to draw meaningful insights from the approximately 1,200 individual submissions to the National Telecommunications and Information Administration’s request for comment on AI accountability.
The comments themselves were overwhelmingly negative. Worries about job loss and copyright featured much more than concerns about discrimination or uncontrollable systems – a curious contrast to the priorities of many AI experts.
Our findings led us to two policy recommendations: (1) developers and policymakers need to recognize that model evaluations do not necessarily generalize and (2) policymakers must demand a sufficient level of evaluation transparency for critical applications.
We share our overall methodology and a study of our evaluations here.
The individual submissions to the NTIA’s AI Accountability RFC give us a window into how people – rather than organizations – perceive the effects of AI. They differ from the organizational submissions in substance and style, tending to be less formal, structured, or prescriptive. Instead, they give us access to perspectives that would be otherwise hard to access at scale.
Our goal in this project was to accurately and concisely summarize these submissions.
Combing through this many comments is hard work. We were curious what a regulatory agency could learn from an analysis that used a large language model (LLM) to reliably draw meaningful insights from a set of comments this large and diverse.
Our first impulse was to ask the LLM outright to tell us the concerns described in the comments, but found that a yes-no question format was more reliable and unlocked a more rigorous type of analysis. However, confidence in the results turned out to be slippery at first.
LLMs can be unreliable, but so too can people, and simply comparing LLM and human answers was insufficient. We ultimately created two evaluation sets – a “gold” set using our colleagues to answer the questions for a sample of the comments and a much larger crowd-sourced set using the same approach – to see where agreement was greatest among answers. We used this to iteratively develop both the prompts for the LLMs, as well as to refine the questions that we were asking, until we arrived at a set of questions and answers in which we could feel relatively confident.
Our experience evaluating a relatively simple analysis yielded two particular lessons, which we explain in greater detail below:
- Developers and policymakers need to recognize that model evaluations do not necessarily generalize
- Policymakers must demand a sufficient level of evaluation transparency
Concrete worries about AI, today
The comments themselves expressed pessimism and worry about the state of AI. Given the nature of an RFC process, self-selection bias certainly explains some of this. But it is still striking how much the concerns differ from what many AI experts prioritize.
Pessimism reigns
Most comments expressed pessimism about the future of AI, while only a smattering expressed optimism. That pessimism seems to come from fear rather than experience: while 9% of comments describe a personal harm, two thirds describe a harm that a group has experienced due to AI.
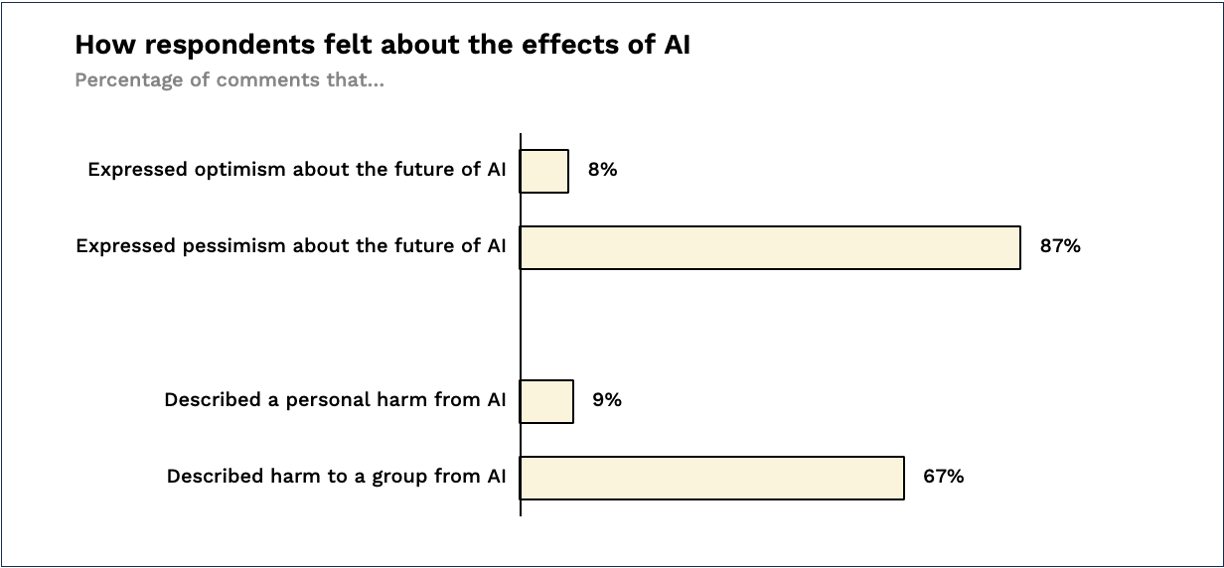
Intellectual property and personal economic impacts top the list of concerns
Intellectual property and personal economic impacts dominated the list of concerns. Curiously, other issues that are important to AI experts – like deepfakes, misinformation, and values alignment – get mentioned but with significantly less frequency. Somewhat surprising, at least to us, was to see bias and discrimination so far down the list.
This does not mean that the commenters do not care about these issues – only that they raised them with less frequency than others.
It is also worth pointing out that this was a request for comment from the Department of Commerce, and so concerns about incomes and intellectual property seem at least thematically appropriate.
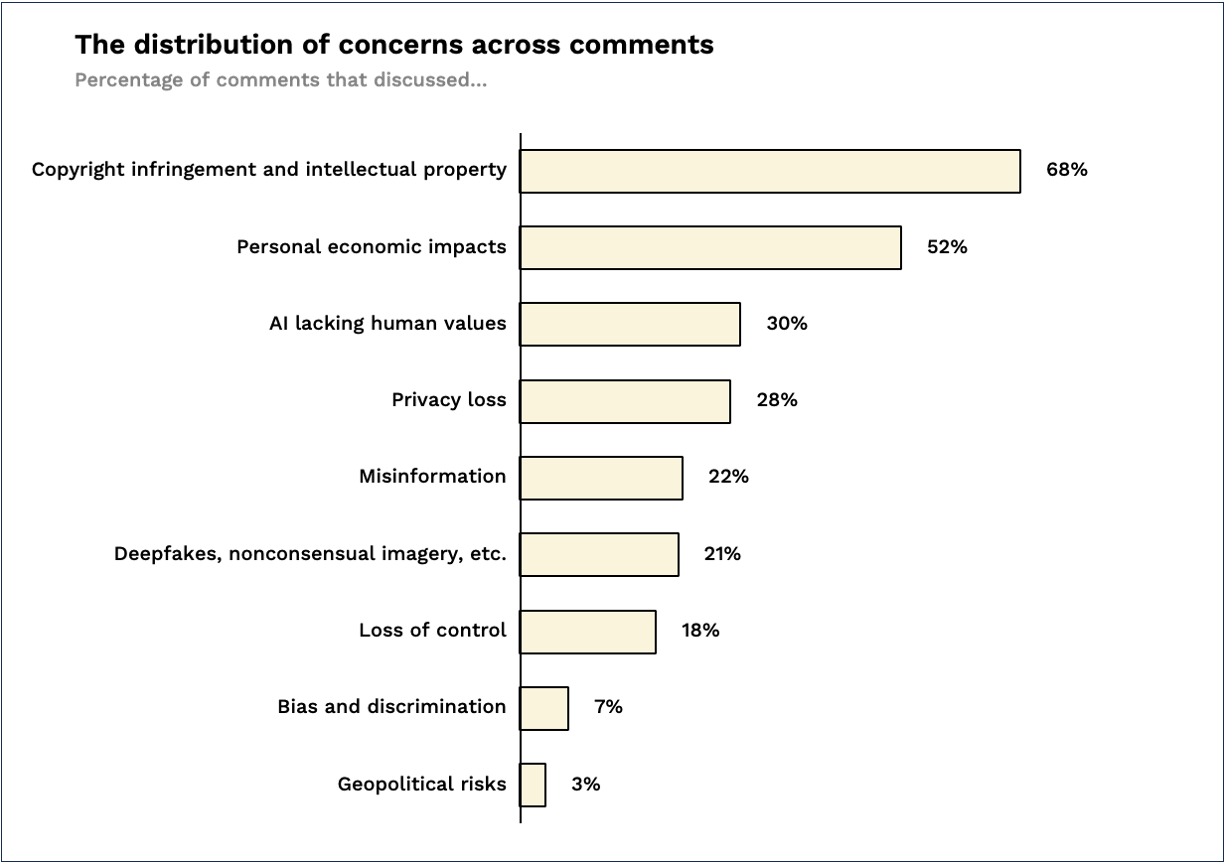
Although artists are only 1.6% percent of the U.S. workforce (according to the Bureau of Labor Statistics), 21% of commenters described themselves as artists. For the most part, their concerns are similar to those of the overall set of commenters, but the intensity of their worry over personal economic impact is much higher.
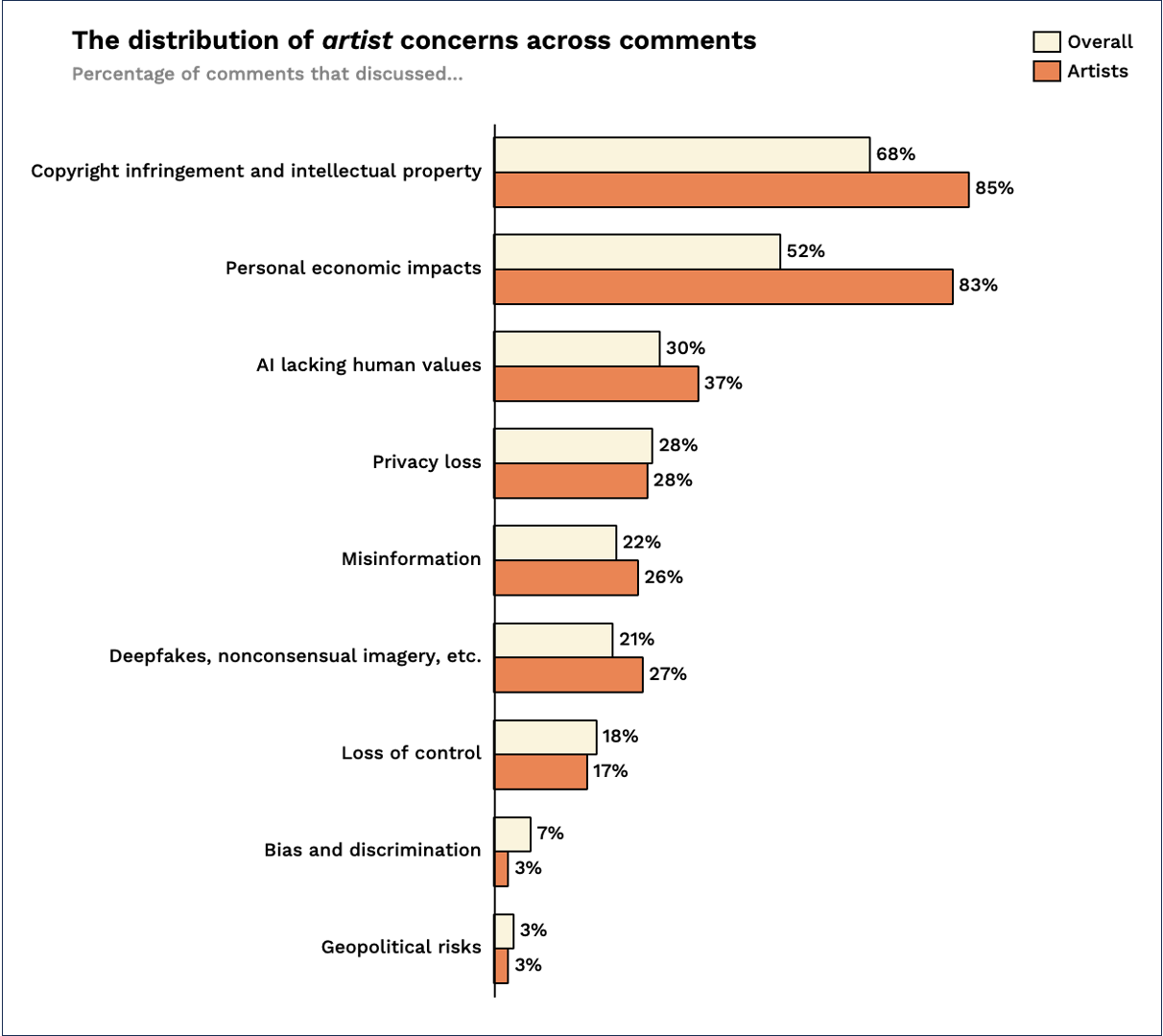
Desire for regulation is high
The individual submissions tend to be lighter on solutions and more pronounced on harms. However, three quarters called for AI regulation. It was interesting to us to see that only 16% thought that regulating AI would be difficult.
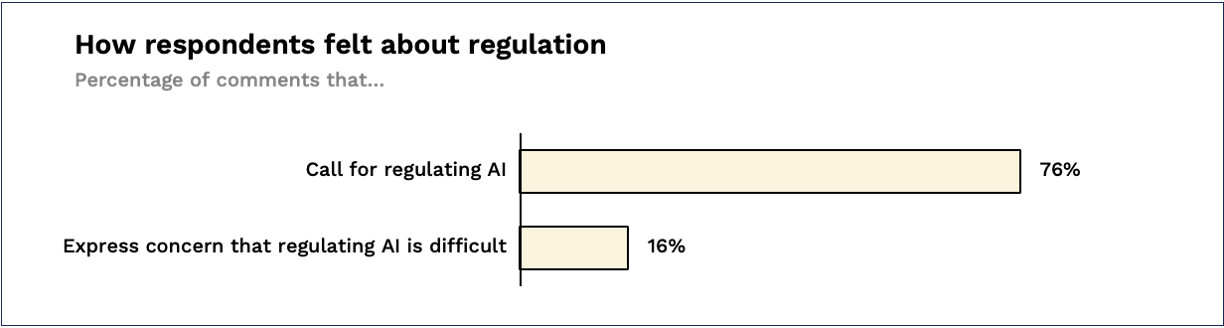
The challenges of rigorous evaluations
While the developers of large language models have posted impressive results on a variety of benchmarks, it is worth remembering: evaluations only tell us how a model performs for a given dataset.
Consider an evaluation about weather in certain locations. If the dataset exclusively covers Pittsburgh, PA, the model might perform similarly for Sub Saharan Africa, but we have no guarantee that the results generalize this way.
The practical implication is that developers and users need to evaluate and validate models for their own uses. Metrics from another dataset can suggest the possibility of similar performance, but without acquiring new data and asking the right questions there is no way to be sure.
There are two parts to an effective evaluation: what it covers (the dataset) and how the results get measured (the metrics).
An evaluation dataset needs to be tailored to the use case and broad enough to cover most reasonable use cases. Being completely exhaustive may not always be possible, especially for large or complex domains, but the more comprehensive the dataset the greater the utility.
The metrics need to provide justified confidence that the model can answer these questions correctly. To do that, developers need to start with goals that are specific and useful. If a weather evaluation metric was whether the model could predict the day’s temperature to +/- 50 degrees Fahrenheit, it would probably be near-perfect; it would also be useless. Getting those questions right is necessary but hard work for developers: they need to understand what they are trying to accomplish and to test rigorously to see if that actually happens.
For our own analysis, whose methodology we comprehensively explain here, we started with an initial set of questions and created test sets (“gold” and crowd-sourced human responses) to compare with the LLM. We then iteratively refined both the questions we were asking, as well as the way we were using the LLM to provide answers, while continually evaluating the agreement between LLM and human answers.
This iterative process was valuable. It was immediately clear that we needed to refine our question list. Some were too ambiguous while others introduced confusion – e.g., we had originally grouped privacy loss and misuse of data as related concepts, but found that by focusing on privacy loss alone we got more reliable answers. We also realized that the model had trouble with outlier inputs – e.g., comments that used graphics or were in a language other than English – something particularly relevant to public-facing applications.
Surprisingly, we also learned that many of our prompting strategies were less helpful than anticipated; in the end, a simple question-answer format proved the most reliable.
This rigorous approach to evaluations leads us to two recommendations:
-
Developers and policymakers need to recognize that evaluations of language models do not necessarily generalize. Unless you specifically evaluate for the use cases that your LLM-based application covers, you have no guarantee that it will work correctly. But effective evaluations are non-trivial: they require sound datasets, well formed evaluation metrics, and appropriate evaluation methodologies. Anything else creates false confidence in a broken process.
-
Policymakers must demand a sufficient level of evaluation transparency for critical applications. The conditions that we describe for effective evaluations can be subverted by opaque reporting and misleading benchmarks, and there are strong incentives for developers trying to bring subpar products to market to do that. We already have an accountability deficit with products like the COMPAS recidivism algorithm; sound regulation could prevent and reverse such failures.
It is worth noting that transparency alone is not a panacea. The substance, completeness, and enforcement of a disclosure regime will determine whether it is effective; this requires explaining the contents of both the dataset and the metrics used. Separately, open source evaluation sets are valuable for good faith actors but can be used to “cheat” on performance metrics if they are added to a training set.
In the case of our analysis of the individual NTIA submissions, our hope is that reviewers will trust the results of our analysis because of the rigor and openness of our evaluation methodology. It is our recommendation that policymakers make this type of approach mandatory where the stakes are sufficiently high – such as for government and adjacent applications.
The trust imperative
If the number of self-identified artists alone is anything to go by, the individual submitters to the NTIA’s AI Accountability RFC are likely a skewed representation of broader society. But that’s not a reason to dismiss what they have to say. In fact, the urgency and trepidation expressed in their comments ought to be motivation to develop sound regulation for AI and to ensure that these rules are made in a way that earns the public’s trust – so future snapshots like this one can tell a very different story.
A powerful way to establish that trust is to demand rigor in the use of evaluations and reporting metrics. Benchmarks and standardized evaluations are one way of ensuring that models work as expected and to build confidence in their abilities. But for that trust to be soundly earned, these evaluations need to be done correctly, reported openly, and not used to justify use beyond the scope of what is truly being measured by the benchmark, e.g., performance on a particular dataset.